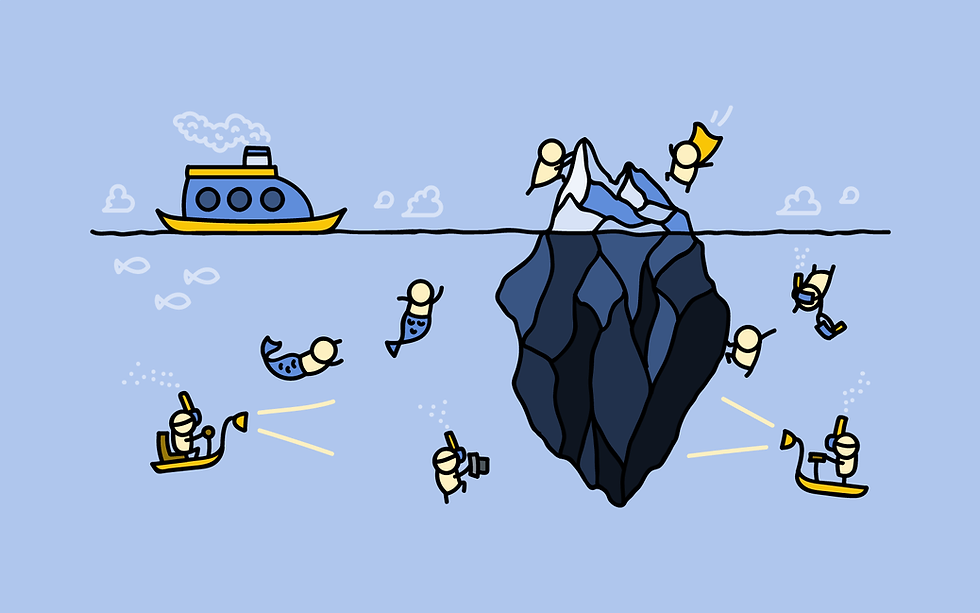
In terms of methodology behavioural science, amongst many other fields of study, has greatly benefited from developments in data science. With the applications of big data, artificial intelligence (AI), machine learning (ML) and many other approaches, we have never had more insights.
However, as these insights progress us into knowing more, I am starting to get the feeling that it is simply more of the same thing, and therefore not necessarily moving us ahead in terms of actual insights. The insights you have are only as good as the data you have; which is based on the measures you collected, and the questions you asked. What wasn’t asked, or measured, isn’t in the data.
What we need is a different style of measurement or question asking, not necessarily more data.
I would like to mention that I’m not against the alignment of behavioural sciences with data science. Not at all. I think it’s a great development, I just don’t think it’s going to get us “all the way”.
Keep in mind, the behavioural sciences are a lot of different disciplines studying human behaviour. Behavioural science is, at best, an umbrella term. Lately, there’s been a focus on getting more and more data, to be able to better see what has happened in the past, in real-time and from there, make predictions about what likely is to continue happening. Neatly segregated by consumer type, demographics, user type etc. Although it seems as if we’re getting to know more about the individual as a whole, what we’re really doing is to gather a lot of data about someone’s behaviours. This type of data, or methodology, does not tell us anything more about any specific individual’s mental processes. It doesn’t tell us their motivation. Their why. And sometimes it doesn’t even tell us their how.
So before we go all starry eyed for behavioural data science, of which I am a fan, we should not forget about our more qualitative approaches to the studying of human behaviour.
Imagine that you have access to someone’s entire transaction history, from all of their financial institutions. That’s a lot of data. Even better, that data is complete (because it’s from all their institutions). You’d know a lot about what’s going on in their life financially. You can see what they purchase, how much they save, maybe even what they’re saving for if their savings account has a name (like “retirement savings” or “wedding budget”). You can deduce the percentage of their post-tax wage they dedicate to savings, if they save at all. You can see their debts if they have them, and how they repay them. From all this quantitative data you can derive a lot. Except for people’s actual situations. Because what looks good on paper, may be hell in real life.
I read the US financial diaries (USFD) and have mentioned them several times before on this blog. The name itself reveals the methodology: they are a diary studies. For this specific studies it meant that participants (households) would account for all their transactions over a specified time period. Not just the easy to track transactions that show up in your banking app (if you use one), but everything else as well. Think of all transactions made in cash and tit-for-that – transactions which were entirely devoid of money changing hands. And when all those transactions are collected, ask the household more about their actual financial situation; for each transaction, ask why. Why was additional income or a windfall not used to repay debt but to double the expense on groceries from normal? Why are households co-holding (having both low interest savings and high interest debt)? Finding out people’s perceptions of their situation, their attitude towards money and their actual living situation. Two people with the same “data profile” might be experiencing their situation drastically differently. One might be feeling mentally well, capable of repaying their debts, managing their money, living their best life. The other, again with the same “profile”, is 3 transactions away from a mental collapse, feels completely overwhelmed by their debt and utterly incapable of managing their finances. You need a method that digs deeper to find that out. And those methods tend to be qualitative in nature.
Now qualitative methods have suffered a bit of a diminished reputation, mostly by the hands of quantitative researchers. Qualitative methods are supposedly too “wishy-washy”, not representative enough (small samples due to the high intensity/duration of the research), and difficult to scale. And whereas some of this is true, the majority of it isn’t.
Like its quantitative counterpart, qualitative research has also been impacted by the Open Science movement, which requires transparency and replicability (mostly done through pre-registration and publishing of all materials used). So qualitative studies can just as easily be replicated, making them a lot less “wishy-washy”. There are also solid theories on the conducting of this method, which can be found in the fields of ethnography and philosophy. Not exactly two fields to sneeze at. So that argument is just void.
Last, and maybe most surprisingly, qualitative methodologies are becoming increasingly easier to scale, because of developments in technology, as well as the data sciences (I know right?!). Technology has allowed us to collect observations easier, as well as store and document them increasingly faster and more accurately. Even for qualitative data. Recording interviews, filming people going through their day and then having them react to it gives us a wealth of information. A lot of user testing (UX and CX) dedicates a significant chunk of its research to these methods.
Now that we have a wealth of more qualitative knowledge, what do we what do we do with it? Analyze it of course! And this is where data science comes in. Developments in sentiment analysis and natural language processing (NLP) through methods such as BERT (Google), TaBERT (Meta), roBERTa (initially Facebook, now Meta) have changed the game for how well we can derive meaningful and deep insights from text (through deep learning NLP). No it doesn’t beat actually sitting down and listening to someone’s story, but it’s a much more scalable approach for sure, also allowing to an even larger extent for the data collector and analyst to be separated (which is recommended for most qualitative approaches – with an eye towards bias).
There we have it. Behavioural science has seen a lot of developments lately. Data science is thriving, it’s just that we might need to amp up the type of data we are collecting. More qualitative approaches and insights are required to really get to the core of our own behaviour, and the mental processes that lie behind them. Some people say that data is everything. But data isn’t everything. Data is only as good as its method. So by that reasoning, method is everything.
For those of you who are becoming increasingly interested in behavioural data science, my own alma mater, Warwick Business School, now offers a course on Behavioural Data Science. So check that out if you think that this may be the direction you’re heading on 😊
top free vr porn sites
There was once a blind man who had so fine a sense of touch that,
when any animal was put into his hands, he could tell what it was merely by
the feel of it. One day the cub of a wolf was put into his hands, and he was asked
what it was. He felt it for