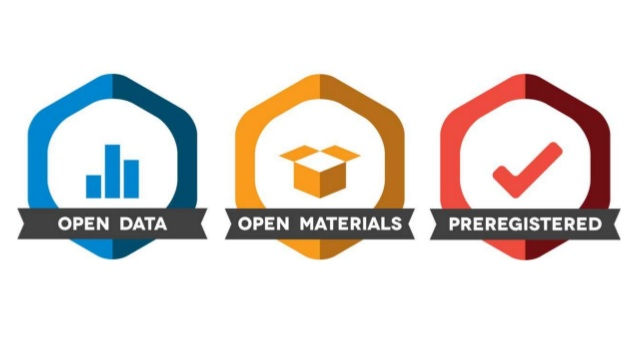
It’s the current bane of my life. I genuinely would not be surprised if the pre-registration for my second study, which is a big data analysis, will have taken three months so far. And that’s just after actually having opened an OSF (Open Science Framework) project.
And that’s not counting the discussions, the understanding the data, the variable creation and testing and the general graphing and analyses run in the 1% sample to see if the data shapes up (or not…). In that case, you might want to add another 6-12 months.
You might be wondering: why am I putting myself through this? Well, first of all, it’s my supervisors who are training me to see this as the norm, so I do quietly resent them (only a bit). But second, and most importantly, because it’s seen as good science. Let’s dive into that argument.
Arguments for pre-registration A multitude of fields, psychology most notably, have run into a replication crisis. As in, some of the most established effects did not replicate. Effects that academics have built their careers on. Effects that companies have built their strategies on. Effects that governments have built policies on. They didn’t replicate. They might not even exist. Oops.
Now, there is a multitude of reasons why a result might not replicate:
Different sample sizes It is possible that the initial study was done on a small sample and when testing it on a larger sample, the effect disappears. That is possible, depending on the size and robustness of the effect. It can work the other way around as well: the initial study tested a massive group and found an effect yet the smaller group you’re testing doesn’t display it, or too a much weaker effect. This can just be a main characteristic of the population you’re testing, although it isn’t super likely, given that the effect actually exists. Lastly, you might have tested different populations altogether. It sounds a bit odd for an exact replication, but it is often seen when testing different age groups, socio-economics backgrounds, nationalities/culture or even gender. Some effects hold for one subgroup (say teenagers) but not for the other (pensioners). That does happen, and these are factors that need to be taken into account.
Methodological differences The last point in the section on “Different sample sizes” can be argued to be a methodological difference. Afterall, the method often specifies a sample. Or at least, the published paper should give a researcher enough information about the sample to replicate it. But, there can be smaller, more insidious differences in methodologies. In experiments where there’s interaction between experimenter and participant it might be whatever is said (the script) that leads to a much larger effect that if that script is not properly adhered to. If the script also isn’t provided, anyone who wants to replicate the study will have great difficulties doing so. Other methodological differences can be found in the surroundings, the direct setting, the amount of people a participant interacts with, whether an experiment is done late or early in the day, effects can even be seasonal (no joke). All this information needs to be given, but you try finding all this in the methodology of an experiment paper. You might be hard-pressed to find it. Just in general, as a quick exercise, next time you read the methodology of a paper, ask yourself whether the information given is enough to make you conduct an exact replica of the study. Amuse me. For third party data analysis, or just big data analysis (it can be your own data), there are different issues. There is no method of conducting, but all of the method focuses on the analysis. This is where the replication crisis had a field day: it found p-hacking. Let’s dive into that in the next section.
Improper Science To continue on the previous section: you can p-hack a study. This misreporting or data mining for results, or selecting out subgroups and different variables until anything significant is found is often referred to as “p-hacking”, the p standing for the probability value assigned to indicate whether a finding is likely to occur under a null hypothesis (null hypothesis significance testing in statistics). If only the significant results are reported for subgroups that worked, and all the rest is muffled away in an appendix (if you’re lucky) or simply never reported, how are you supposed to know? You can’t. This is bad science, but is has happened for quite a while. If you have no access to the raw data, nor the data files (assuming a written statistical language) than how are you supposed to see what is actually going on? Some “academics” and I’m using that term with disdain, have run analyses on one subgroup, found significant results, and reported them as true for the whole population tested. That isn’t science, that’s bullsh*t. You might be asking yourself: isn’t this blatantly obvious in the paper itself? The answer is often no. If you have no access to the raw materials, how are you going to determine what’s right, and what isn’t? Pre-registration is seen as a way around this.
So, there are good arguments to make for why you should pre-register a study. If you have your data (and you are allowed to look at a small percentage of your sample 1-10%, but do need to indicate that) and adhere to the analysis plan you have made beforehand, you are a good scientist!
How do you pre-register? There are a few sites, or projects and frameworks as they often call themselves, that allow you to pre-register a study. Examples of these are Open Science Framework (OSF) and the Centre for Open Science (COS).
When you go onto these sites, you create a project, give it a snazzy name and invite your collaborators so everyone can see and add to it. Once you have a proper plan de campagne, you can upload a bunch of files (plan of analysis, list of variable names, coding scripts, surveys, outline of method (if conducting an experiment), short summary of literature etc.) and once finished you can lock this in. This sounds really easy, but coming up with those takes several months, if not longer.
Once locked in, you are able to add files (tables showing results from the planned analysis, raw data), but you can’t edit anything. That’s the good stuff!
Also important to note: the default of these projects is to be private, until you “lock them in.” This locking in actually publishes the study on the site. So OSF has now published your pre-registration, and everyone with an OSF account (or just everyone, I’m not too sure) can now see it. This means that when submitting your paper to a journal, you will also have to provide the pre-registration link. This is so reviewers can see whether the study you’ve done is actually up to scratch, but it’s also a determinant in whether your submission will even be accepted.
Some journals have gone as far as to say that you need a pre-registration or one hell of a reason as to why you didn’t pre-register your study. And to be quite frank, that’s not an argument I’m willing to have, because I believe in good science myself. I also believe in open access, so for all the studies that I’m allowed to, I will also publish the raw data. This is more difficult to do when the data is from a third party, however.
What if your plan doesn’t work? Now, let’s say you’re a good scientist. You’ve worked months on the pre-registration, then run the experiment, then load in the data and run the analysis scripts you’ve so nicely prepared. And nothing works. Brilliant. This does happen. I have run into this issue in the first study I did in my PhD. Can you imagine my horror?
We had a great plan for analysis, but it assumed a normal distribution of responses to a variable (recall error measured in pounds). It didn’t follow a normal distribution at all. It was massively positively skewed (it was just zero inflated) and the analysis wouldn’t work. In the end, we created a different variable called “probability of correct recall” which was a binary variable indicating whether you recalled your expenditure correctly or not (0/1). This wasn’t in the pre-registration, however.
When it comes to changing your pre-registered analysis, transparency is key. You’ll have to acknowledge in your result section that the initial analysis you ran (and specify which one that was!) didn’t work and you’ll have to outline all the reasons for why it didn’t. As such, you’ll take those reasons to justify a different approach and only then present the results from this approach. After that, it’s fine. This really isn’t that uncommon. Two lessons learned in one pre-registration.
It is also possible that your data came from a third party, as such, the scenario above is a bit less likely. Why? Because third party data always needs to be checked to see whether it has been collected successfully (to the extent that it’s useful to you), whereas you need to lock a pre-registration before you conduct an experiment, because the method needs to be locked in as well. With third party data, to be sure, a 1-10% samples often get checked before committing to a pre-registration.
I’m currently in that process myself. I’m looking at a 1% sample of the data. Initially, to see whether all the variables worked and measured what they were supposed to be measuring (they weren’t). Then, to see how the data was shaped (normal distribution, skewed etc.), and of course found a lot of the variables I created to be zero inflated. I mean, why wouldn’t they be? But knowing all this, you have to change your plan of analysis. Although don’t forget to mention that you’ve looked at a 1-10% sample before locking in the study! If you fail to mention this, you’ll likely be accused of p-hacking, even if you had the best intentions!
Overall, pre-registering is a b*tch of a process. But, if you ask me, it’s the only way forward. No more shady business. Openness, transparency and replication will show what our field is made of. Which effects hold and we can build on, and which just don’t and have been massively inflated or p-hacked to death.
We might end up redeveloping the whole damn field we’re in. Are you joining the revolution?
Comments